Apple's On-Device model performs better than Microsoft's Phi-3-mini, Server model comparable to GPT-4 Turbo
3 min. read
Updated on
Read our disclosure page to find out how can you help MSPoweruser sustain the editorial team Read more
Key notes
- The race to develop more efficient, capable AI models ultimately leads to better products, more intuitive user experiences, and a broader range of AI-powered tools at our fingertips.
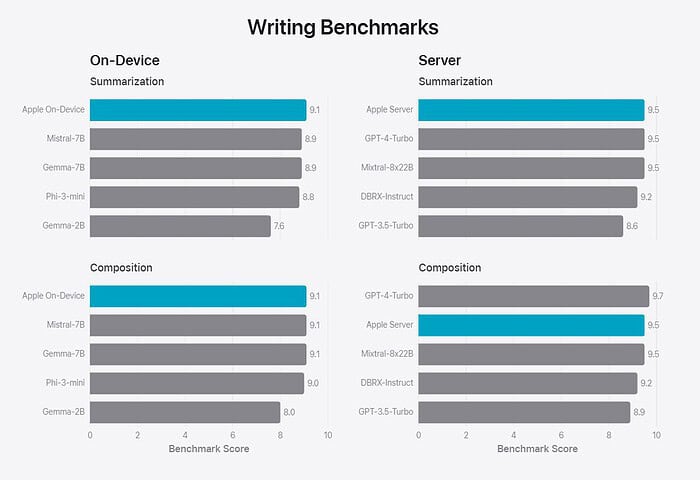
In April this year, Microsoft announced Phi-3 family of small language models (SLMs). The Phi-3-mini with a 3.8 billion parameter language model is trained on 3.3 trillion tokens and it beats Mixtral 8x7B and GPT-3.5. Microsoft’s recently announced Copilot+ PCs which use large language models (LLMs) running in Azure Cloud in concert with several of Microsoft’s world-class small language models (SLMs) to unlock a new set of AI experiences you can run locally, directly on the device.
At WWDC 2024, Apple announced Apple Intelligence (its Copilot alternative) for its devices. Apple Intelligence is powered by multiple highly capable generative models. Similar to Microsoft’s approach, Apple uses both On-Device models and server-based models. Yesterday, Apple detailed two of the foundation models it is using to power Apple Intelligence.
- Apple On-Device model is a 3 billion parameter SLM
- Apple Server is a LLM hosted on Apple’s own Private Cloud Compute and running on Apple silicon servers
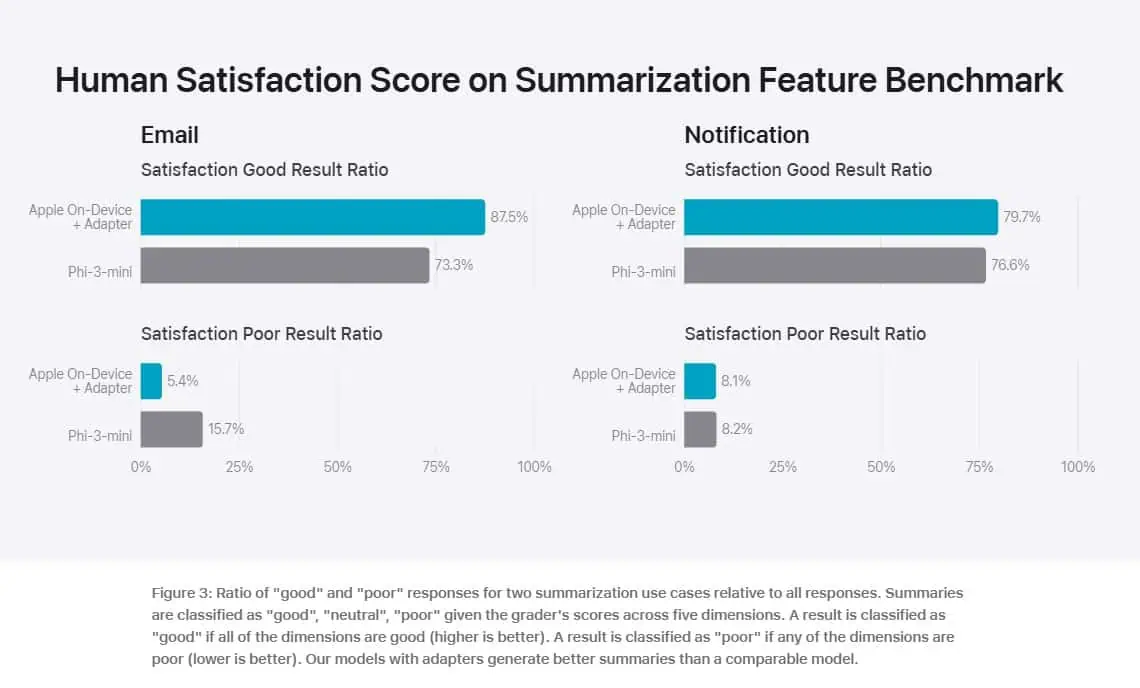
Technical Details:
- Apple’s foundation models are trained on Apple’s AXLearn framework.
- Apple trained the models with high efficiency and scalability on various training hardware and cloud platforms, including TPUs and both cloud and on-premise GPUs.
- Apple trained its foundation models on licensed data, including data selected to enhance specific features, as well as publicly available data collected by our web-crawler, AppleBot.
- The on-device model uses a vocab size of 49K, while the server model uses a vocab size of 100K, which includes additional language and technical tokens.
- On iPhone 15 Pro, Apple was able to reach time-to-first-token latency of about 0.6 millisecond per prompt token, and a generation rate of 30 tokens per second.
To evaluate the performance of these models, Apple compared them with both open-source models (Phi-3, Gemma, Mistral, DBRX) and commercial models of comparable size (GPT-3.5-Turbo, GPT-4-Turbo). Apple claims that its models were preferred by human graders over most comparable competitor models. For example, the Apple on-device model with 3B parameters, outperforms models including Phi-3-mini, Mistral-7B, and Gemma-7B. And the Apple server model compares favorably to DBRX-Instruct, Mixtral-8x22B, and GPT-3.5-Turbo while being highly efficient.
You can check out the Apple’s evaluation results below.
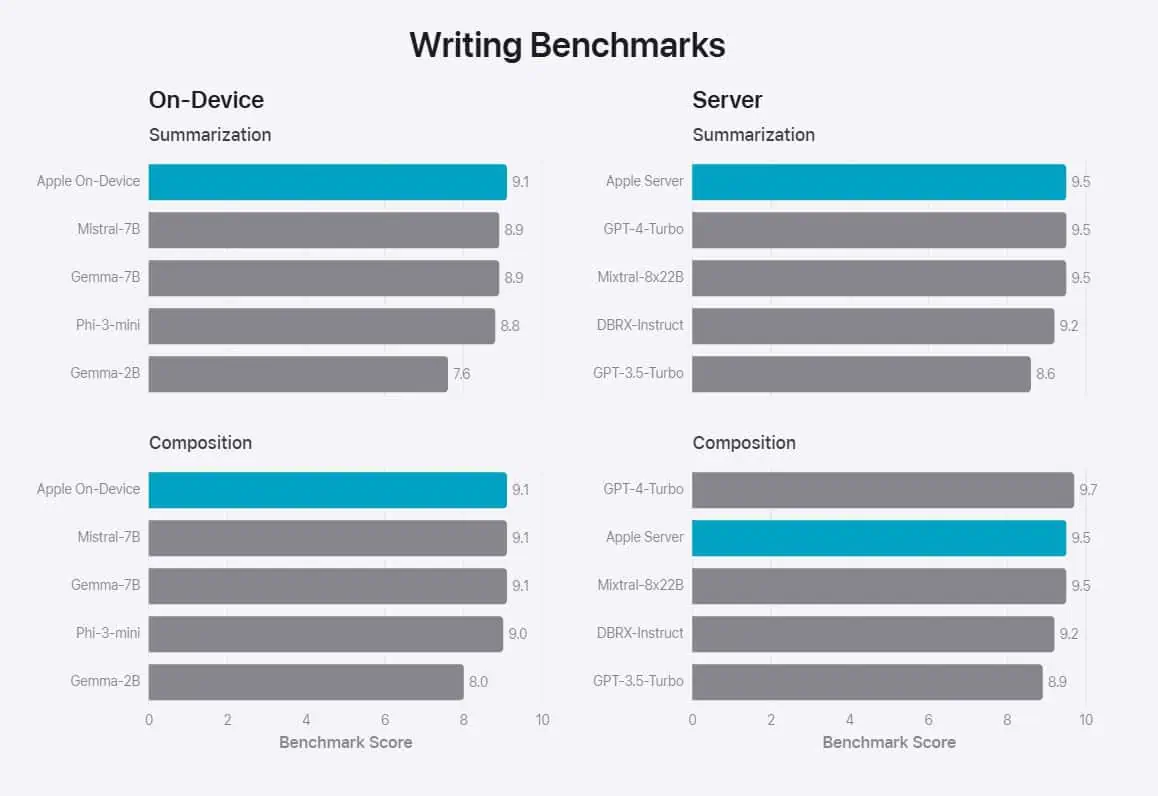
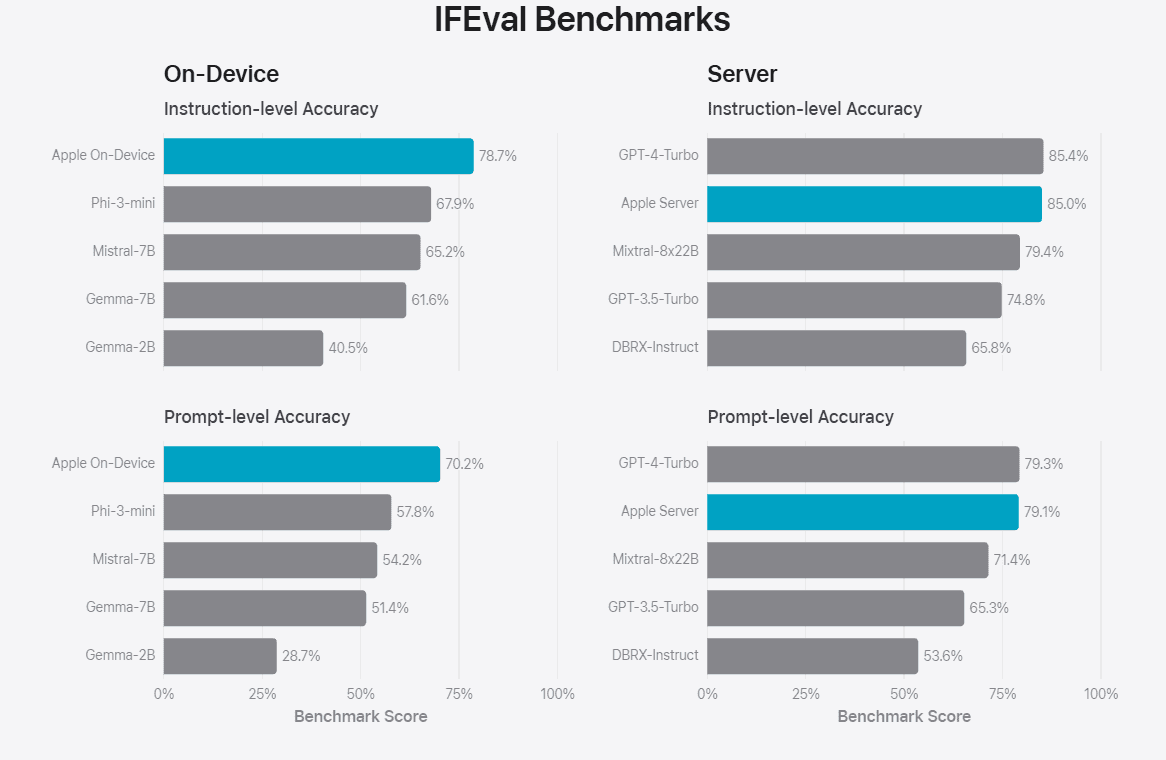
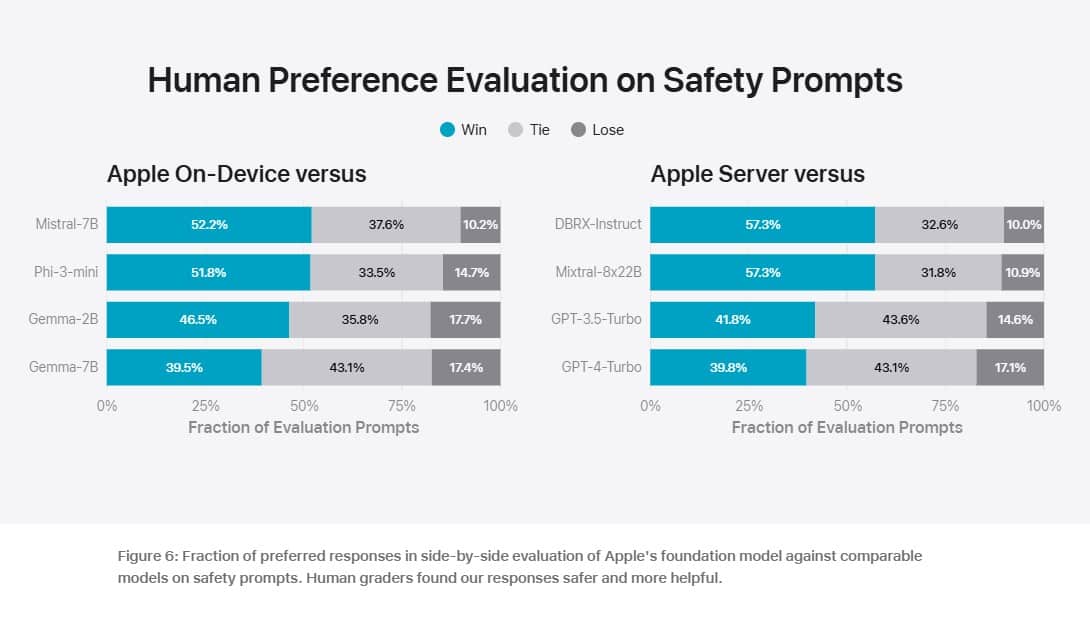
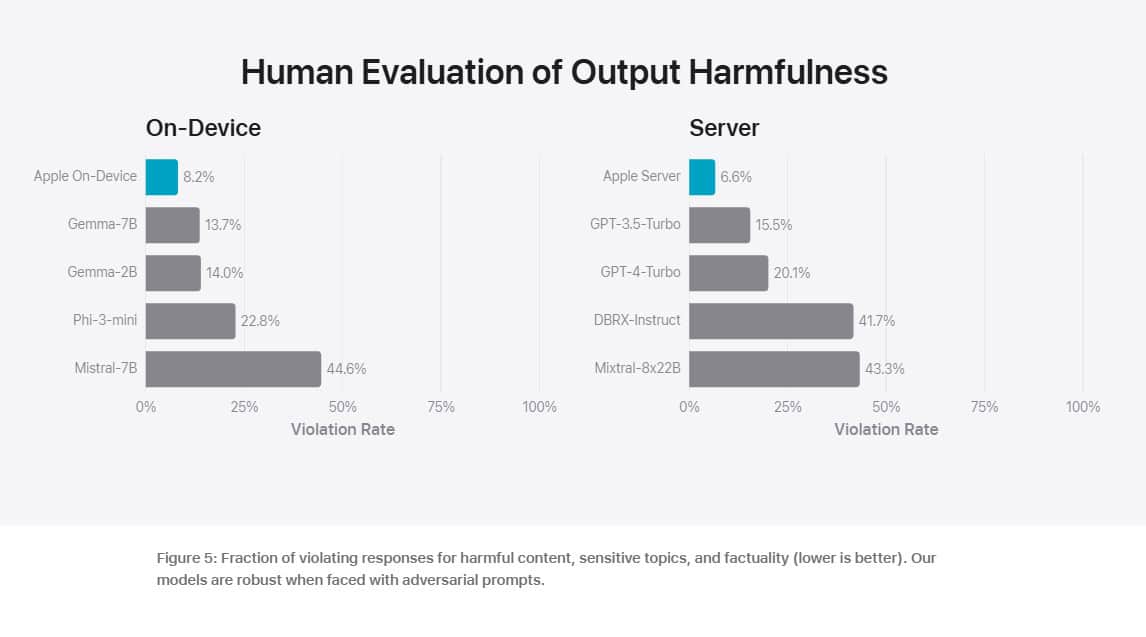
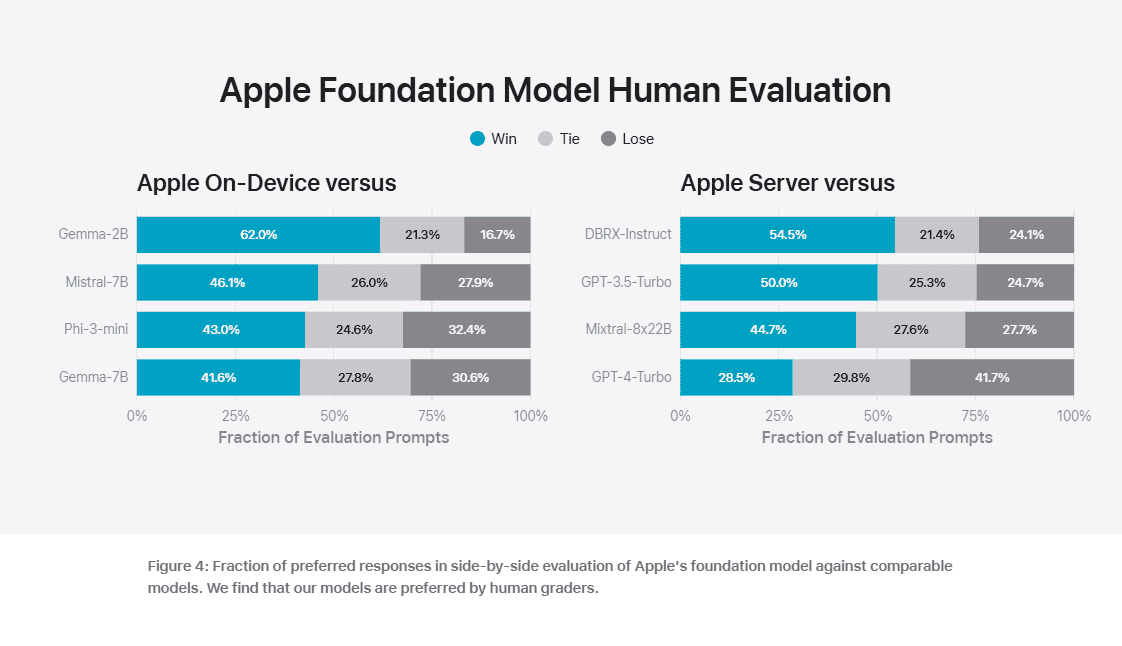
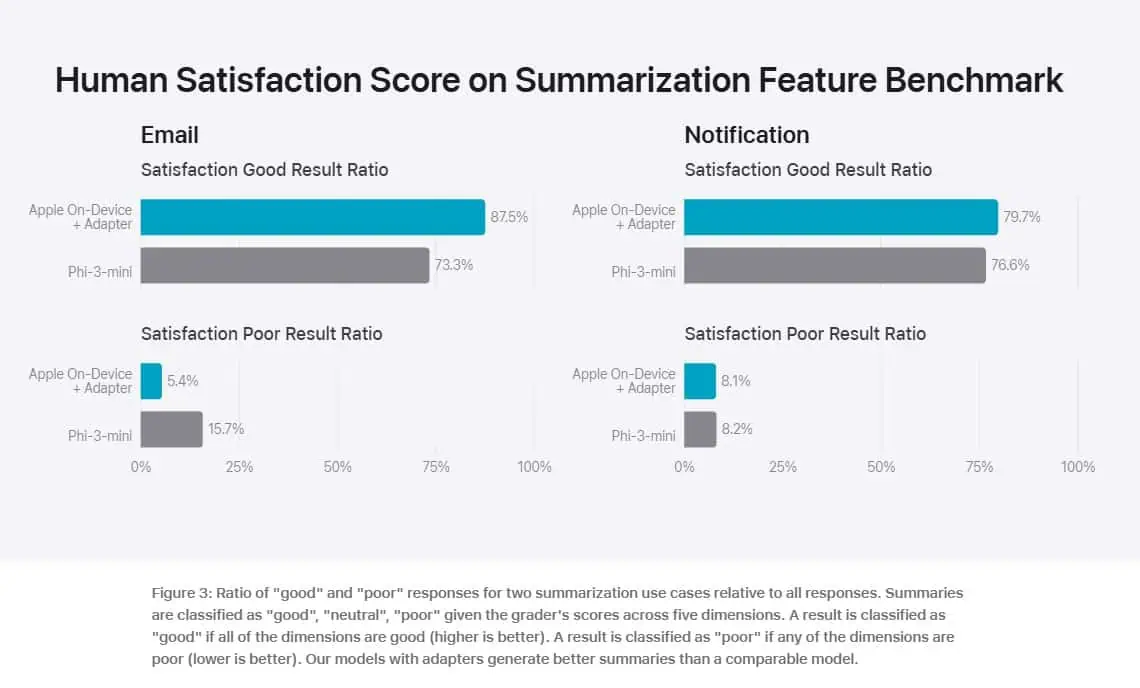
Apple’s strategic use of both on-device and server-based models mirrors Microsoft’s approach, indicating a trend towards hybrid AI solutions that balance power and efficiency. The impressive performance of Apple’s models, particularly their speed and preference among human graders, underscores the potential for smaller, more efficient models to rival larger ones in certain applications.
As these two giants continue to innovate, the future of AI looks increasingly promising. Consumers can expect to see more powerful, personalized AI experiences integrated into their devices and workflows. Whether it’s Microsoft’s Copilot+ or Apple’s Apple Intelligence, the convergence of AI and everyday technology is set to redefine how we interact with the digital world.
User forum
0 messages